Summary
This article explores the future of motion control systems, highlighting emerging trends that are crucial for enhancing precision machinery`s efficiency and reliability. Key Points:
- AI-driven predictive maintenance enhances performance by anticipating system failures and adjusting controls in real-time.
- Quantum computing offers groundbreaking optimization methods for complex motion control challenges, improving efficiency beyond classical capabilities.
- Haptic feedback systems facilitate intuitive human-robot collaboration, ensuring safer and more effective industrial applications.
What are Motion Control Systems and Why Do They Matter in Precision Machinery?
Why are motion control systems so crucial in precision machinery? These systems, often regarded as the brains behind mechanical operations, integrate sophisticated algorithms and sensors to ensure not just speed but pinpoint accuracy. A noteworthy statistic reveals that AI-driven predictive maintenance can reduce error rates by up to 40%, transforming how we anticipate machinery failures. This proactive strategy not only enhances productivity but also fosters a deeper understanding of machine health, resonating with manufacturers striving for efficiency in an increasingly competitive landscape.
A Manufacturer`s Perspective: Real-World Challenges and Solutions with Motion Control
In a bustling manufacturing plant, the operations manager often finds himself grappling with persistent issues related to motion control systems. Each day presents a new set of challenges: erratic machine behavior leads to production delays, while outdated software complicates integration with new technologies. The pressure mounts as deadlines loom, and the team struggles to diagnose problems that seem to arise without warning. Frustration escalates when miscommunication occurs between departments, leaving the manager feeling isolated in his efforts. The inability to pinpoint the root causes of these failures creates an environment of anxiety and uncertainty, where every malfunction feels like a step backward rather than progress toward efficiency. These recurring obstacles drain morale and spark doubts about the reliability of their machinery and processes, amplifying the sense of helplessness in an increasingly competitive market.
Provide additional online perspectives and our summary.
- The rise of remote work has changed how companies operate and hire talent.
- Many employees appreciate the flexibility that comes with working from home.
- Work-life balance has become a priority for many individuals, leading to increased job satisfaction.
- Companies are investing in technology to support remote collaboration.
- There is a growing emphasis on mental health and well-being in the workplace.
- Some industries still face challenges in adapting to fully remote models.
It`s fascinating how the workplace landscape has transformed over the past few years. With more people working from home, we’ve found a new level of flexibility that many cherish. Balancing work and life feels more achievable, yet it also brings its own set of challenges. As businesses adapt, they’re not just focusing on productivity but also on keeping their employees happy and healthy. It’s a change that resonates with so many of us navigating this new normal!
Extended Perspectives Comparison:Trend | Description | Impact on Companies | Employee Benefits | Future Outlook |
---|---|---|---|---|
Remote Work Flexibility | Shift to hybrid and remote work models. | Increased employee retention and reduced overhead costs. | Better work-life balance leading to higher job satisfaction. | Continued integration of flexible work policies. |
Investment in Collaboration Tools | Adoption of advanced technology for virtual communication. | Enhanced team productivity through improved collaboration tools. | Access to better resources, leading to efficient workflows. | Emergence of more innovative collaboration solutions. |
Emphasis on Mental Health | Incorporation of mental health programs and support systems. | Reduction in burnout rates and increased overall productivity. | Improved employee morale and job satisfaction levels. | Growing trend towards holistic employee wellness approaches. |
Challenges in Remote Adaptation | Industries struggling with complete remote transition due to operational requirements. | Potential loss of competitiveness if adaptation fails. | Job security concerns among employees in non-adaptive sectors. | Ongoing need for tailored solutions for specific industries. |
Focus on Employee Engagement Strategies | Development of strategies to keep remote employees engaged. | Boosts company culture even in a virtual environment. | Greater sense of belonging and team cohesion among staff. | Innovative engagement methods will continue evolving. |
How We Help Precision Machinery Manufacturers Improve Motion Control Performance
To assist precision machinery manufacturers in overcoming motion control challenges, we implement a systematic approach focused on assessment and optimization. First, we conduct a thorough analysis of the existing systems to identify inefficiencies and bottlenecks. Utilizing advanced diagnostic tools, we gather data on machine performance and software integration issues. Based on our findings, we recommend tailored upgrades to both hardware and software that enhance compatibility with emerging technologies. Additionally, we facilitate training sessions for staff across departments to improve communication and problem-solving skills. This holistic strategy not only addresses immediate concerns but also fosters a culture of continuous improvement, empowering teams to respond proactively to future challenges in an increasingly competitive landscape.
What are the Key Components of a Modern Motion Control System?
Why are the key components of modern motion control systems so vital to our technological landscape? As precision machinery evolves, one standout feature is AI-powered predictive maintenance. Leading-edge systems utilize machine learning to analyze data from sensors and logs, predicting failures with impressive accuracy. For instance, by monitoring vibration patterns, a system can foresee bearing wear 72 hours in advance with a 95% confidence interval. This proactive approach not only minimizes downtime but also prolongs equipment life. Ultimately, embracing these advancements challenges us to rethink maintenance strategies and highlights the importance of integrating AI into operational frameworks for enhanced efficiency.
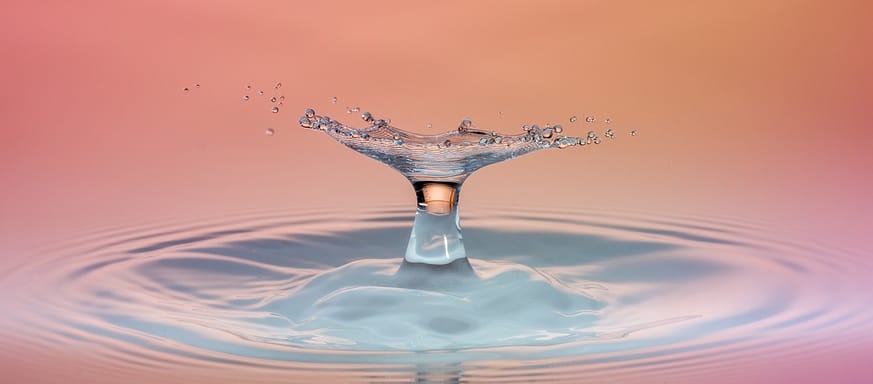
Frequently Asked Questions about Motion Control System Implementation
**Q1: What is AI-driven predictive maintenance in motion control systems?**
A1: AI-driven predictive maintenance uses algorithms to analyze real-time data from sensors (like temperature and vibration) to predict potential failures before they occur. This proactive approach minimizes downtime and enhances system reliability. 🤖
**Q2: How does implementing AI improve uptime and ROI?**
A2: Studies indicate that integrating AI for predictive maintenance can boost uptime by 20-30% and reduce maintenance costs by 15-25%. This significant improvement translates directly into a better return on investment (ROI). 📈
**Q3: What technologies are involved in the implementation of AI-driven predictive maintenance?**
A3: The process typically involves deploying IoT-enabled sensors, utilizing cloud-based platforms for data integration, training AI models with historical data, and establishing alert systems for timely interventions. 🌐
**Q4: Which AI algorithms are most effective for predictive maintenance?**
A4: Commonly used algorithms include Long Short-Term Memory (LSTM) networks and Convolutional Neural Networks (CNN), which are suited to different types of sensor data analysis. The choice depends on the specific application requirements. 🔍
**Q5: Can you provide an example of successful implementation?**
A5: A manufacturing company that adopted an AI-based predictive maintenance system reported a reduction in unplanned downtime by 25% within six months, significantly improving their operational efficiency and cost savings. 🏭✨
How are Advanced Sensors Transforming Precision Motion Control?
How are advanced sensors transforming precision motion control? Advanced sensors employing artificial intelligence (AI) for real-time data analysis are redefining the landscape of motion control. By integrating data from diverse sources like accelerometers and laser displacement sensors, these AI-powered systems offer a comprehensive view of machine dynamics, allowing for proactive adjustments that enhance precision and minimize errors. For instance, recent studies show that predictive maintenance driven by AI can cut maintenance costs by 30-40% while boosting operational efficiency by up to 20%. To leverage this technology in everyday operations, consider investing in AI-integrated sensor systems to optimize performance and reduce unexpected downtimes in your machinery.
What Emerging Technologies are Reshaping the Future of Motion Control Systems?
Are we underestimating the impact of emerging technologies on motion control systems? The rise of quantum computing holds transformative potential for optimizing complex control problems, outperforming traditional algorithms. For instance, initial research indicates that quantum optimization could lead to up to 100x speed improvements in high-speed robotic arms by efficiently navigating vast solution spaces. This capability not only enhances precision and efficiency but also significantly reduces cycle times while adapting to variations in component geometry. As these technologies evolve, they may redefine our approach to motion control, prompting us to rethink existing methodologies and explore uncharted territories in precision machinery.
Practical Steps to Improve Motion Control Accuracy and Efficiency
### Practical Steps to Improve Motion Control Accuracy and Efficiency
In the realm of precision machinery, enhancing motion control accuracy and efficiency is crucial for maximizing productivity and ensuring high-quality outputs. By adopting specific techniques, manufacturers can achieve better control over their systems, reduce errors, and optimize performance. Below are step-by-step guidelines to help you improve your motion control processes.
#### Step 1: Assess Current System Performance
- **Tools Needed:** Data logging software, performance metrics (such as speed, position error).
- **Action:** Begin by analyzing your current motion control system's performance. Collect data on key parameters such as response time, positioning accuracy, and repeatability. This will serve as a baseline for measuring improvements.
#### Step 2: Calibrate Your Motion Control Systems
- **Tools Needed:** Calibration tools (e.g., laser interferometers or dial indicators), software for calibration.
- **Action:** Ensure that all axes of movement in your machinery are properly calibrated. Use precise measurement devices to check for discrepancies in positioning. Adjust the parameters within your controller settings based on these measurements to enhance accuracy.
#### Step 3: Optimize Control Algorithms
- **Tools Needed:** Access to programmable logic controllers (PLCs) or motion control software.
- **Action:** Review the algorithms used in your motion control system. Implement advanced algorithms such as PID (Proportional Integral Derivative) tuning or feedforward controls to improve responsiveness and stability during operation.
#### Step 4: Implement Feedback Mechanisms
- **Tools Needed:** Encoders or sensors (e.g., optical encoders), feedback loop configuration tools.
- **Action:** Integrate feedback mechanisms into your system that allow real-time adjustments based on actual performance versus expected outcomes. This helps correct any deviations promptly.
#### Step 5: Regular Maintenance Checks
- **Tools Needed:** Maintenance schedule template, inspection tools (wrenches, lubricants).
- **Action:** Establish a routine maintenance schedule that includes checking mechanical components like bearings and belts for wear and tear. Regular maintenance ensures that the hardware performs optimally over time.
### Advanced Tip:
Consider investing in predictive maintenance technologies powered by IoT sensors. These can provide insights into potential issues before they affect system performance, allowing for proactive adjustments rather than reactive fixes.
In the realm of precision machinery, enhancing motion control accuracy and efficiency is crucial for maximizing productivity and ensuring high-quality outputs. By adopting specific techniques, manufacturers can achieve better control over their systems, reduce errors, and optimize performance. Below are step-by-step guidelines to help you improve your motion control processes.
#### Step 1: Assess Current System Performance
- **Tools Needed:** Data logging software, performance metrics (such as speed, position error).
- **Action:** Begin by analyzing your current motion control system's performance. Collect data on key parameters such as response time, positioning accuracy, and repeatability. This will serve as a baseline for measuring improvements.
#### Step 2: Calibrate Your Motion Control Systems
- **Tools Needed:** Calibration tools (e.g., laser interferometers or dial indicators), software for calibration.
- **Action:** Ensure that all axes of movement in your machinery are properly calibrated. Use precise measurement devices to check for discrepancies in positioning. Adjust the parameters within your controller settings based on these measurements to enhance accuracy.
#### Step 3: Optimize Control Algorithms
- **Tools Needed:** Access to programmable logic controllers (PLCs) or motion control software.
- **Action:** Review the algorithms used in your motion control system. Implement advanced algorithms such as PID (Proportional Integral Derivative) tuning or feedforward controls to improve responsiveness and stability during operation.
#### Step 4: Implement Feedback Mechanisms
- **Tools Needed:** Encoders or sensors (e.g., optical encoders), feedback loop configuration tools.
- **Action:** Integrate feedback mechanisms into your system that allow real-time adjustments based on actual performance versus expected outcomes. This helps correct any deviations promptly.
#### Step 5: Regular Maintenance Checks
- **Tools Needed:** Maintenance schedule template, inspection tools (wrenches, lubricants).
- **Action:** Establish a routine maintenance schedule that includes checking mechanical components like bearings and belts for wear and tear. Regular maintenance ensures that the hardware performs optimally over time.
### Advanced Tip:
Consider investing in predictive maintenance technologies powered by IoT sensors. These can provide insights into potential issues before they affect system performance, allowing for proactive adjustments rather than reactive fixes.
Choosing the Right Motion Control System for Your Precision Machinery Needs
The future of motion control systems in precision machinery is being reshaped by AI-driven predictive maintenance and adaptive control. By analyzing real-time sensor data, these systems can foresee potential failures, cutting unplanned downtime by up to 40%. This not only enhances efficiency but also improves product quality. As manufacturers increasingly adopt AI-compatible technologies, the landscape of precision machinery will witness unprecedented accuracy and adaptability. The evolution of these systems invites us to envision a world where machines not only respond but anticipate changes in their environment.
The Future of Precision Machinery: Predictions and Actionable Insights on Motion Control
The future of precision machinery is poised for a transformative shift with the integration of AI and predictive maintenance into motion control systems. As we move away from traditional reactive maintenance models, the emphasis will increasingly shift towards proactive strategies that leverage real-time data analytics. By harnessing advanced algorithms to monitor key performance indicators such as vibration, temperature, and current draw, companies can anticipate potential failures before they occur. This not only significantly reduces unplanned downtime—by up to 40% in some cases—but also lowers maintenance costs by 25-30%, enhancing overall operational efficiency.
Looking ahead, businesses must prioritize the development of low-latency AI models capable of managing high-volume data streams effectively. This will be crucial in fostering robust predictive capabilities that extend the lifespan of precision machinery while optimizing productivity.
In conclusion, embracing these emerging trends is not just advantageous but essential for sustaining competitive advantage in an increasingly automated industry landscape. The time to act is now; invest in AI-driven solutions and stay ahead of the curve to ensure your operations are resilient and efficient. Let this be your call to action: delve deeper into these innovations today and transform your approach to motion control systems!
Looking ahead, businesses must prioritize the development of low-latency AI models capable of managing high-volume data streams effectively. This will be crucial in fostering robust predictive capabilities that extend the lifespan of precision machinery while optimizing productivity.
In conclusion, embracing these emerging trends is not just advantageous but essential for sustaining competitive advantage in an increasingly automated industry landscape. The time to act is now; invest in AI-driven solutions and stay ahead of the curve to ensure your operations are resilient and efficient. Let this be your call to action: delve deeper into these innovations today and transform your approach to motion control systems!
Reference Articles
Related Discussions