Summary
This article explores the crucial role of AI in safeguarding Industry 4.0 from emerging cybersecurity threats, emphasizing innovative approaches that go beyond traditional methods. Key Points:
- Contextual AI enhances industrial cybersecurity by integrating operational technology (OT) and IT security data to create a comprehensive threat model, moving beyond simple anomaly detection.
- AI-driven Zero Trust architecture continuously verifies every device and user within Industry 4.0 networks, adapting security policies based on real-time risk assessments.
- Federated learning enables organizations to collaboratively train AI models while preserving data privacy, enhancing collective defenses against cyber threats.
Exploring AI's Role in Cybersecurity for Industry 4.0
This article will delve into how AI is shaping the future of cybersecurity in Industry 4.0, discussing key tools, advantages, challenges, and future trends in AI-driven cyber defense. In this context, specific methodologies such as machine learning algorithms, anomaly detection techniques, and deep learning frameworks will be highlighted. It will also mention the types of data sources utilized, such as IoT device telemetry and network traffic patterns, and emphasize the importance of integrating real-time threat intelligence. Together, these elements promote the development of adaptive security measures that can keep pace with emerging threats, thereby enhancing the resilience of smart manufacturing environments against cyber attacks.
Identifying the Unique Security Challenges of Industry 4.0
However, this technological evolution introduces unique security challenges. For instance, implementing a Zero Trust Architecture can enhance security in decentralized environments characteristic of Industry 4.0. Moreover, specific materials used in IoT devices may present vulnerabilities due to firmware limitations or reliance on outdated protocols. The role of machine learning algorithms also becomes crucial; their ability to detect anomalies can adapt to emerging threats effectively. Understanding these complexities is essential for navigating the cybersecurity landscape within Industry 4.0.
Key Aspects | Details |
---|---|
Transformation in Industry 4.0 | Integration of AI, IoT, and cyber-physical systems enhancing operational efficiency. |
Security Challenges | Unique vulnerabilities due to interconnected systems and reliance on outdated protocols. |
AI`s Role in Cybersecurity | Real-time analysis using machine learning for anomaly detection and threat identification. |
Predictive Analytics | Anticipating attacks by analyzing historical data; tools like SparkCognition exemplify this approach. |
Automated Response Systems | AI-driven tools autonomously neutralizing threats, such as CrowdStrike Falcon for endpoint detection. |
Understanding How AI Solutions Tackle Industry 4.0 Threats
In light of Industry 4.0's digital landscape, there is a pressing need for AI-driven cybersecurity solutions capable of managing extensive data volumes, adapting to new threats, and autonomously orchestrating responses. These solutions utilize various machine learning principles, such as supervised and unsupervised learning techniques, to detect anomalies in real-time data streams effectively. By analyzing diverse datasets—including sensor information and network traffic—these systems can identify potential security breaches.
Moreover, feature engineering plays a crucial role in enhancing detection accuracy by optimizing the relevant attributes extracted from raw data. Implementing successful case studies where AI has been deployed in industrial contexts can further illustrate its effectiveness against emerging cybersecurity threats within Industry 4.0 environments.
Leveraging Anomaly Detection to Enhance Security
Take Darktrace as an example. This AI tool leverages machine learning techniques to detect and respond to threats by creating a "pattern of life" for each user, device, and network, then using this baseline to identify anomalies. Additionally, when designing these systems, specific algorithms such as Isolation Forest or Long Short-Term Memory (LSTM) networks can be considered to improve the accuracy of anomaly detection. The importance of adjustable parameters—such as threshold sensitivity, the diversity of training data, and real-time monitoring capabilities—cannot be overlooked. Moreover, integrating these functions with existing Security Information and Event Management (SIEM) systems can offer a more comprehensive perspective, ensuring that anomaly detection better complements the overall cybersecurity strategy in Industry 4.0 environments.
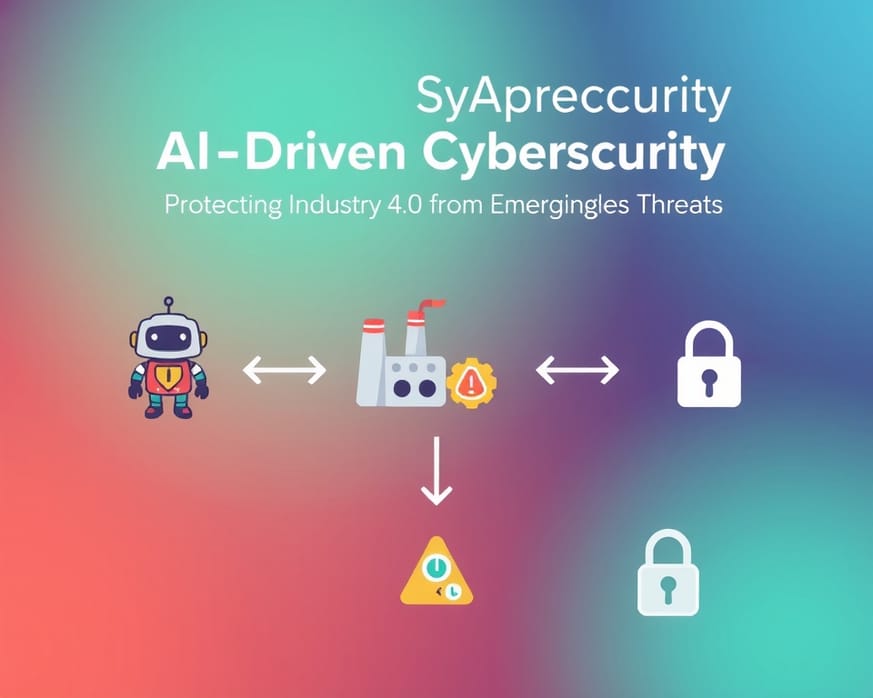
Utilizing Predictive Analytics to Prevent Attacks
Incorporating specific algorithms like machine learning techniques—such as decision trees or neural networks—can significantly improve the accuracy of these predictions. The importance of real-time data ingestion from IoT devices cannot be overstated; various data types, including network traffic and user behavior patterns, contribute critical insights for more precise threat forecasts. Additionally, effective feature engineering plays a vital role in refining model performance by tailoring parameters that align with unique operational contexts in Industry 4.0 environments.
Implementing Automated Responses for Swift Threat Mitigation
To enhance automated responses for swift threat mitigation, machine learning algorithms can be employed to predict and detect threats as they occur. Integrating threat intelligence feeds ensures access to up-to-date information on emerging vulnerabilities. Additionally, customizable response protocols should be developed based on specific industry risks and compliance requirements. Tools like Security Orchestration, Automation, and Response (SOAR) platforms are essential for streamlining incident management processes effectively.
Creating Self-Healing Systems for Continuous Protection
In the context of Industry 4.0, AI-enhanced cybersecurity offers several benefits, including significantly faster detection and response times. This capability helps prevent damage and limits downtime in critical industrial processes by quickly addressing threats as they arise. The integration of advanced sensors and IoT devices plays a crucial role in enabling real-time monitoring and self-repair functions within these systems, further bolstering their effectiveness against emerging risks.
Recognizing the Advantages of AI-Driven Cybersecurity Solutions
Key Technologies Empowering AI in Cyber Defense for Industry 4.0
1. Natural Language Processing (NLP) for Threat Intelligence: NLP allows AI to analyze vast amounts of text-based information, including threat reports and cybersecurity articles, to detect emerging threats and identify potential vulnerabilities. By employing advanced algorithms such as machine learning and deep learning techniques, organizations can achieve real-time threat detection. Moreover, utilizing sophisticated data analytics helps uncover patterns within large datasets, aiding in the prediction of possible security weaknesses. Incorporating examples of current AI tools or platforms would provide practical insights into their application in the field, while emphasizing customization options could illustrate how these solutions are tailored to address specific cybersecurity needs across different industries effectively.
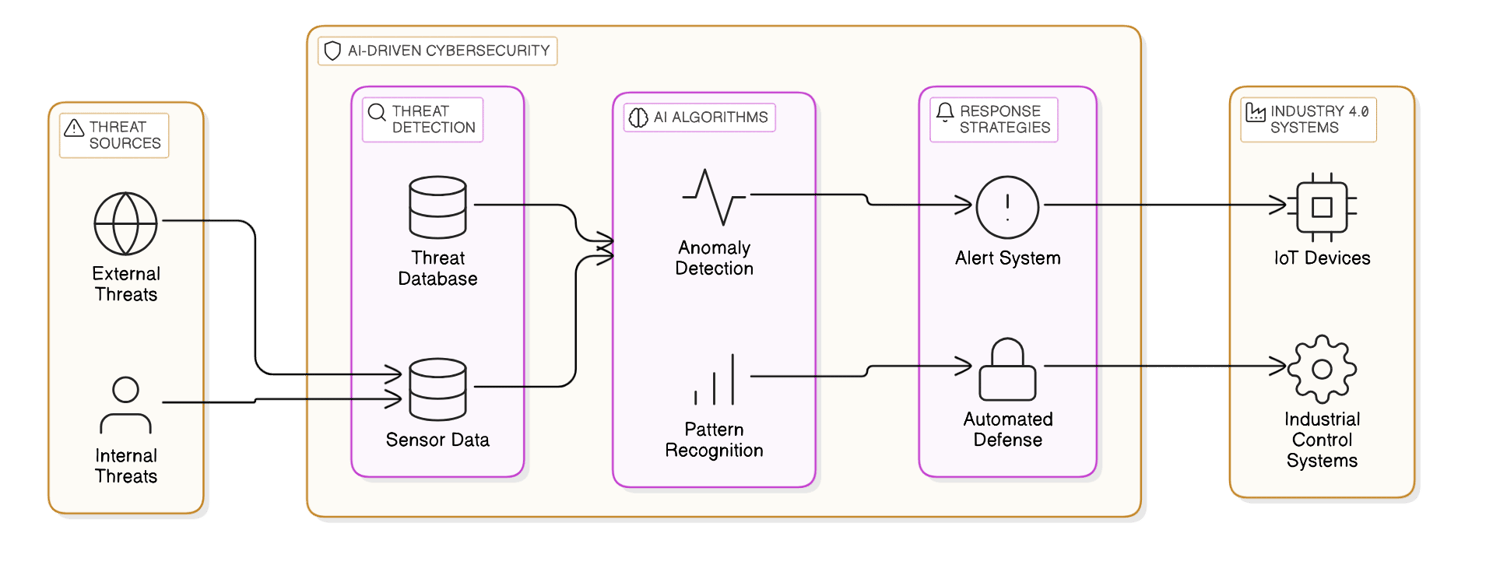
Examining Key Technologies Supporting AI in Cyber Defense
Moreover, incorporating algorithms like reinforcement learning can further enhance threat detection capabilities. The role of big data analytics is also significant in processing large volumes of security-related data to spot patterns that signal potential threats. Advances in hardware technology, such as graphics processing units (GPUs), facilitate quicker data analysis while specialized cybersecurity frameworks support the integration of artificial intelligence, collectively strengthening cyber defense strategies in Industry 4.0 contexts.
Anticipating Future Trends and Innovations in AI Cybersecurity
However, implementing AI in cybersecurity within Industry 4.0 presents several hurdles:
1. **High Implementation Costs**: Establishing AI-driven cybersecurity systems demands substantial investments in technology, infrastructure, and expertise.
2. **Data Privacy Concerns**: The extensive data collection required for effective cybersecurity can raise privacy issues; companies must navigate compliance with regulations such as GDPR.
3. **False Positives and Bias**: Machine learning models often generate false positives, mistakenly identifying legitimate activities as threats. Additionally, biases inherent in algorithms can compromise accuracy, necessitating ongoing human oversight.
4. **AI vs. AI Warfare**: Cybercriminals are also harnessing AI to execute sophisticated attacks. This arms race compels defense systems to continually evolve and improve.
Looking ahead at the future of AI in cyber defense for Industry 4.0:
- **Collaborative AI for Cross-Industry Threat Intelligence**: Companies are investigating collaborative AI models that facilitate shared threat intelligence across various sectors. This cooperative approach allows industries to combine resources effectively against global cyber threats.
- **Predictive and Prescriptive Analytics for Cyber Resilience**: Future developments will not only focus on predicting attacks but will also provide prescriptive solutions that guide industrial operators toward proactive resilience strategies.
- **Edge AI and On-Device Processing**: With the proliferation of IoT devices, there's an increasing reliance on edge computing—processing data locally on devices rather than cloud-based solutions—to reduce latency and enhance real-time response capabilities essential for distributed industrial environments.
As Industry 4.0 transforms operational efficiencies and productivity levels, cybersecurity must advance correspondingly. The integration of automation through AI has become crucial for safeguarding interconnected industrial systems driven by data flow. By utilizing tools such as anomaly detection powered by AI, Security Information Event Management (SIEM), and self-healing mechanisms, industries can proactively tackle threats while minimizing response times and bolstering resilience amidst challenges ranging from privacy concerns to adversarial attacks.
The trajectory of cybersecurity in this era hinges on continuous innovation alongside emerging technologies like quantum computing—laying a foundation not just for securing Industry 4.0 but also fostering sustainable growth in an increasingly connected landscape.
Reference Articles
Rethinking Security — AI-Enhanced Automation in Cyber ...
Explore how AI-enhanced automation is redefining cybersecurity for Industry 4.0, providing real-time threat detection, predictive analytics, ...
Industry 4.0 and cybersecurity – How to protect your ...
In this blog, I'll discuss how manufacturers can approach the additional vulnerabilities that come with Industry 4.0 and the interconnectivity it provides.
AI-Driven Cybersecurity: An Overview, Security Intelligence ...
Based on these AI methods, in this paper, we present a comprehensive view on “AI-driven Cybersecurity” that can play an important role for ...
Source: ResearchGate4 AI-powered cyber threats and why AI cybersecurity is the ...
1. Automated phishing campaigns · 2. AI-enhanced social engineering · 3. Zero-day exploits and AI-generated malware · 4. Deepfake attacks.
Source: GcoreAI-DRIVEN CYBERSECURITY THREATS AND ...
The research focused on three key areas: improving protection against emerging threats from artificial intelligence, outlining future AI instruments available ...
Source: CSUSB ScholarWorksAI-Driven Cybersecurity and Threat Intelligence: Cyber ...
Moreover, AI enhances the ability to detect anomalies, predict potential threats, and respond swiftly, preventing risks from escalated. As cyber threats become ...
Source: Amazon.comAI and Cyber Security: Innovations and Challenges
AI's impact on cybersecurity is transformative, providing significant advantages such as enhanced threat detection and response.
Source: eSecurity Planet
Related Discussions