Summary
This article explores the challenges and solutions in implementing Enterprise AI, highlighting its significance across various industries. Key Points:
- Quantifying the ROI of AI is essential for industries like manufacturing and energy, focusing on measurable outcomes such as cost savings and efficiency gains.
- The future of AI architecture combines edge and cloud solutions to address data sovereignty while enabling real-time analytics and model training.
- Explainable AI (XAI) is critical in regulated sectors, requiring frameworks that clarify complex decisions to both technical and non-technical audiences.
The Importance of AI in Today's World
To illustrate the impact of AI further, it’s worth noting specific examples and statistics that underscore its transformative effects across different industries. For instance, in healthcare, AI-driven tools have been shown to increase diagnostic accuracy by substantial margins, leading to better patient outcomes. In manufacturing, predictive maintenance powered by AI can reduce downtime by up to 30%, translating into significant cost savings for organizations.
Moreover, ethical considerations surrounding the deployment of AI technologies are crucial in shaping public opinion. As companies navigate these challenges, they must also focus on emerging trends like explainable AI and sustainability initiatives that address contemporary issues while fostering trust among users.
Overall, the discussions at this year’s event really captured how enterprise-level implementations can move beyond pilot projects into full production environments—making a real difference across numerous fields and ultimately bringing us closer to an era where AI truly is everywhere.
Highlights from Intel Vision 2024
In addition to its impressive specifications, discussions at the event also touched on critical aspects like hardware optimization techniques that contribute to energy efficiency metrics and overall performance benchmarks. The principles surrounding scalability and adaptability of AI models in enterprise contexts were explored too, emphasizing how these innovations can overcome production challenges. Furthermore, the integration of cutting-edge materials—such as silicon photonics—was highlighted for its role in improving data transfer rates within AI infrastructure. These insights collectively provided a more comprehensive understanding of how advancements like Gaudi 3 are shaping effective implementations of AI solutions across various industries.
Key Topic | Insights | Examples/Statistics | Implications | Future Trends | |
---|---|---|---|---|---|
AI Integration in Industries | AI is essential across sectors, improving efficiency and outcomes. | In healthcare, AI tools increase diagnostic accuracy; predictive maintenance in manufacturing can reduce downtime by 30%. | AI transforms processes, leading to cost savings and enhanced decision-making. | Continued growth of AI applications will drive innovation and operational improvements. | |
Transitioning from POC to Production | Challenges include scalability, reliability, and performance optimization. | Case studies emphasize the need for robust data integration and collaboration between teams. | Successful transitions enhance operational efficiency but require strong organizational readiness. | Focus on refining solutions based on feedback will be critical. | |
Edge AI Technology Benefits | Offers real-time responsiveness with low latency for decision-making. | Local processing enhances privacy and reduces bandwidth needs—ideal for manufacturing environments. | Edge AI improves security while ensuring efficient operations without constant internet access. | Hybrid models integrating Edge AI may become standard in industries like energy. | |
Ethical Considerations in AI Deployment | Addressing algorithmic bias and ethical implications is crucial for public trust. | ||||
Innovative Future Directions of AI | Development of self-improving systems that adapt to environmental changes. | Success seen in sound analytics with adaptive retraining strategies. | Minimizing initial data requirements through generative AI will promote wider adoption. | Collaborations between machines and humans will lead to outstanding results. |
Key Challenges in Transitioning AI to Production
## Transitioning from Proof of Concept (POC) to Production: Key Challenges
Shifting from a Proof of Concept (POC) to deploying an AI project on a larger scale presents numerous hurdles. The main purpose of a POC is to showcase the feasibility and potential benefits of an AI solution for a particular use case. This initial phase allows teams to experiment with and fine-tune their AI models, ensuring alignment with intended goals. However, once we move toward full production, our priorities change significantly; now we must concentrate on aspects like scalability, reliability, and performance optimization.
For instance, addressing scalability often involves implementing load balancing techniques or adopting microservices architecture that can efficiently handle increased demand. Furthermore, maintaining high data quality is crucial; this could mean establishing robust methods for continuous monitoring and validation of training datasets throughout the project lifecycle.
Real-world case studies can offer valuable insights into effectively navigating these deployment obstacles. Additionally, fostering strong collaboration between data scientists and IT teams is essential for ensuring seamless integration and ongoing maintenance of AI systems in operational environments.
Understanding Data Integration and Infrastructure Needs
- **Data Integration and Infrastructure:** It's vital to ensure smooth data integration and a sturdy infrastructure. This preparation allows AI systems to accurately interpret and capture all important details. For example, in a Smart City context, merging Internet of Things (IoT) data with weather forecasts and historical information can enhance the ability to anticipate maintenance needs or improve city heating systems. Incorporating ETL (Extract, Transform, Load) processes for data consolidation is also essential, along with exploring cloud-based solutions like AWS or Azure for scalability and flexibility. The use of APIs (Application Programming Interfaces) facilitates seamless communication between different systems, which can help clarify the complexities involved in integration.
- **Organizational Readiness:** Transitioning from pilot projects to full-scale production requires that the organization is prepared as well. Engaging cross-functional teams, refining the solution based on user feedback, and ensuring staff receive adequate training are crucial steps in this process. These practices contribute significantly to embedding the AI solution into everyday operations effectively.
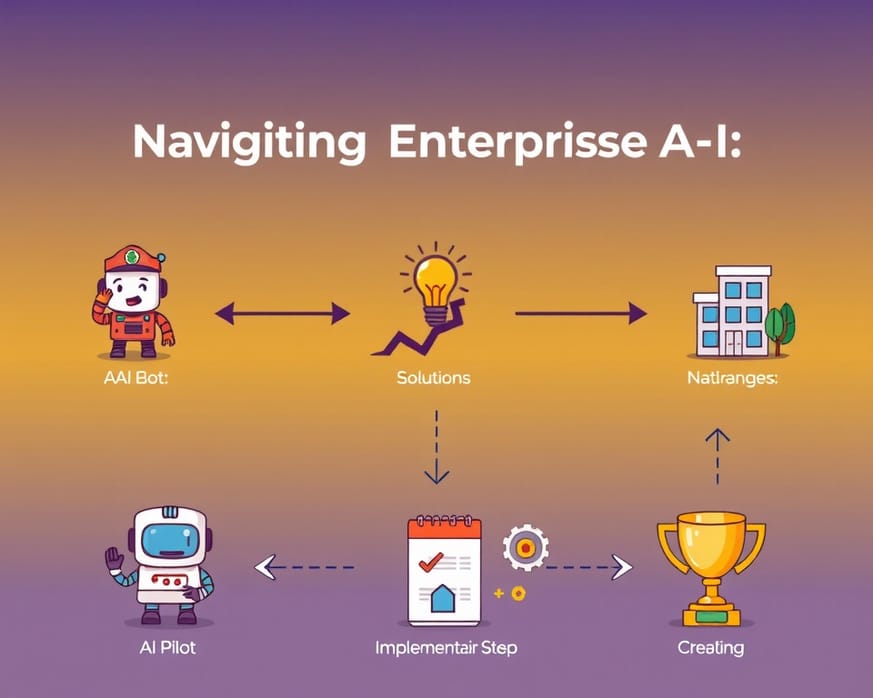
Preparing Your Organization for AI Deployment
**Cloud Solutions:** These options provide flexibility and scalability, making them well-suited for applications that demand significant computational power. They also allow for better accessibility, as long as data privacy and security measures can be effectively managed within the cloud environment.
**Local Infrastructure:** On the other hand, running workloads locally gives organizations more control over their systems and performance. This is particularly beneficial for applications that require strict data privacy, low latency responses, or must comply with specific regulatory standards.
**Hybrid Approaches:** Often, a blend of cloud services and on-premises solutions can deliver an optimal balance—catering to unique business requirements while balancing cost-effectiveness, performance levels, and security considerations.
At [byteLAKE], most of our AI implementations are focused on Edge AI technology. This choice makes perfect sense, especially in manufacturing settings where every moment is crucial. Edge AI brings remarkable benefits in such environments by processing data closer to its source.
Choosing Between Cloud and Local Infrastructure for AI
Benefits of Edge AI in Manufacturing
The Role of Hybrid Solutions in Energy Management
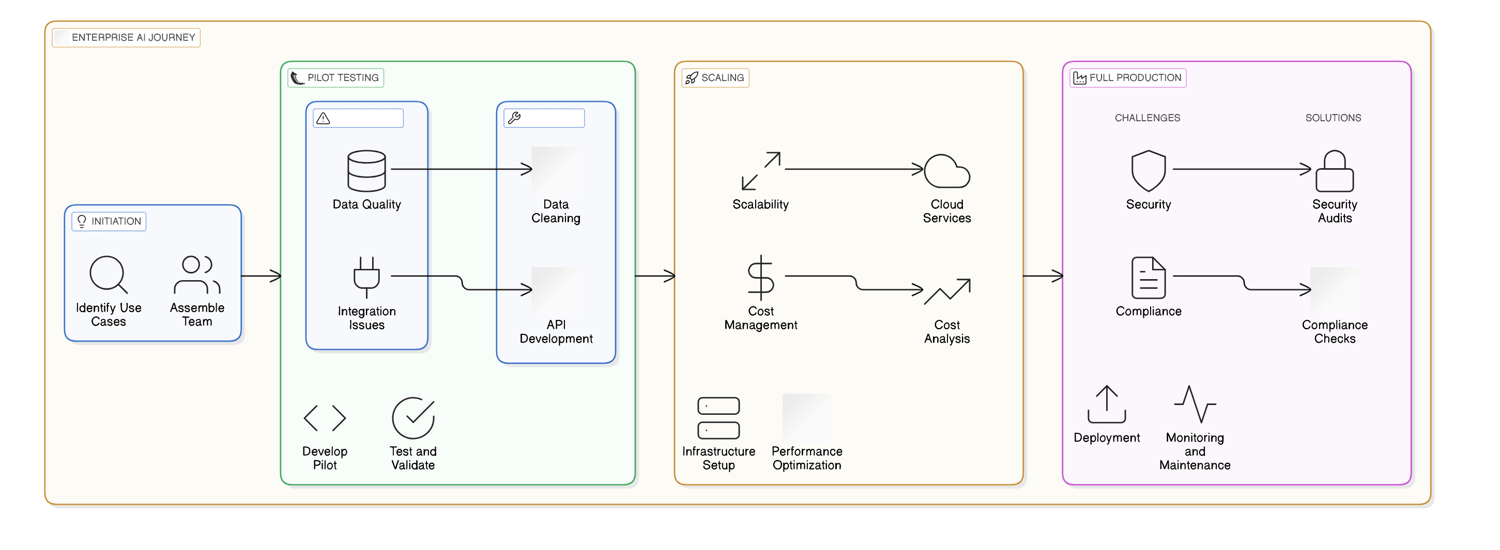
Emerging Trends in AI Adoption and Innovation
The Future of Self-Improving AI Systems
Reference Articles
2024 Enterprise AI Forecast: From Pilot to Production
In 2024, enterprises will increasingly prioritize production-grade AI solutions with a focus on Business Value and Total Cost of Ownership.
Source: AI21 LabsScaling Generative AI: Navigating the Journey from Pilot to ...
Find out how Squirro offer solutions designed to address the realities of scaling generative AI that are practical, precise, and reliable.
Source: SquirroAI pilot to production: challenges & solutions with ...
HPE Private Cloud AI bridges the gap to production with secure, scalable infrastructure, federated data access, and a rich AI software ecosystem.
Launching a Successful AI Pilot Program: A Guide for ...
Continuous monitoring and flexibility are key to navigating the complexities of AI pilots: Establish regular intervals for interim updates to assess progress ...
Source: ScottMaddenFrom Pilot to Production – Deploying Gen AI Applications
The following sections outline key factors to consider as you navigate this transition, ensuring that your organization can fully leverage the power of Gen AI ...
Source: ScottMaddenNavigating the Challenges of Enterprise AI Adoption
The challenge lies in not only recruiting externally for AI talent but also retraining internal resources. Striking a balance between these ...
Source: Finextra ResearchHow to succeed with enterprise AI - HPE Community
However, Forbes reports that fewer than 1 in 10 AI projects get beyond pilot and into production, much less realize return on investment.
The Explosive Growth of Generative AI in Enterprises ...
For enterprises to transition successfully, they need to focus on creating actionable strategies, such as running targeted pilot programs, ...
Source: Medium · Kanerika Inc
Related Discussions