Summary
This article explores how Edge Intelligence and DeepSeek R1 are revolutionizing the Internet of Things (IoT) through real-time AI processing, addressing critical needs in security and efficiency. Key Points:
- Edge intelligence not only reduces latency but also enhances data sovereignty and security, crucial for regulated industries such as healthcare and finance.
- Innovative AI model compression techniques and edge hardware optimization are essential for deploying complex AI models on resource-limited devices, ensuring efficient performance.
- The rise of Web3 aligns with edge computing`s decentralized nature, facilitating secure blockchain transactions and the management of diverse edge device networks.
Understanding Edge Intelligence and Its Importance
The Internet of Things (IoT)—which encompasses our connected smart devices like smartphones, wearables, cameras, and home appliances—has significantly changed how we live our daily lives. Traditionally, these devices operate by sending data to far-off servers for processing and analysis. This is somewhat akin to a student who sends every question to a teacher instead of attempting to think through the answers independently. However, as we introduce an increasing number of connected devices into our environment, this method reveals its shortcomings: delays in response times, issues concerning the privacy of information transmitted over networks, and congested internet connections.
To address these challenges, edge intelligence emerges as a crucial solution. By processing data closer to where it originates—near the device itself—edge computing minimizes latency and reduces reliance on cloud services. This not only optimizes bandwidth usage but also enhances security by limiting the amount of sensitive information traveling across networks. Advanced sensors and energy-efficient processors are often integrated into edge devices, enabling real-time AI processing that can lead to quicker decision-making and more effective resource utilization.
In essence, as IoT continues to evolve with numerous interconnected gadgets around us, adopting edge intelligence principles could greatly improve overall efficiency and responsiveness in various applications.
What Is Edge Computing and How Does It Work?
## Transitioning from Cloud to Edge: A New Approach to Data Processing
Edge computing allows devices to handle data right at the "edge" of the network instead of routing everything back to centralized servers. Imagine empowering students to tackle challenges independently rather than relying solely on their teacher for answers. Edge Intelligence takes this concept further by equipping devices with AI capabilities natively, enhancing their autonomy and efficiency.
This shift not only reduces latency but also saves bandwidth, as data processing occurs closer to where the information is generated. For instance, edge devices such as IoT sensors and gateways play a crucial role in gathering real-time data without overwhelming cloud resources. These devices often utilize low-power processors and advanced communication protocols like MQTT, ensuring they operate efficiently while maintaining connectivity.
Moreover, implementing robust security measures at the edge is essential to protect sensitive data as it travels through various networks. By understanding how these elements come together within edge computing architecture, we can appreciate its functionality and the numerous benefits it offers in today’s digital landscape.
Key Insights | Description | Impact on IoT | Challenges | Future Prospects |
---|---|---|---|---|
Edge Intelligence | Processing data closer to the source reduces latency and enhances security. | Improves efficiency in real-time applications like autonomous vehicles and smart cities. | Requires substantial computing resources despite model compression. | Advancements in AI models and integrated cloud-edge solutions will optimize performance. |
DeepSeek R1 | Utilizes innovative distillation methods to deploy powerful AI on edge devices with limited resources. | Enables sophisticated functionalities on everyday devices, enhancing autonomy. | Compromises may occur in complex reasoning tasks due to model simplification. | Compact devices will execute advanced algorithms effectively as technology evolves. |
Real-Time Decision Making | Local processing allows for instant responses without waiting for cloud communications. | Critical for applications requiring immediate action, such as industrial safety systems. | Integration complexity across numerous edge devices can hinder deployment effectiveness. | Emerging adaptive algorithms will improve analytics capabilities by learning from local data. |
Data Privacy and Security | Sensitive information remains local, reducing exposure during transmission over networks. | Enhances privacy standards across various sectors including healthcare and smart cities. | Robust security measures are essential to protect sensitive data at the edge level. | Focus on developing specialized security protocols tailored for edge environments. |
Scalability of Solutions | Seamless deployment across diverse IoT environments is crucial for widespread adoption of edge intelligence technologies. | Promotes cost-effective operations while maximizing network performance through localized data handling. | Hardware limitations can restrict scalability when deploying advanced AI models uniformly across devices. | Collaboration between cloud services and edge systems will create a more efficient framework for managing IoT workloads. |
Introducing DeepSeek R1 and Its Innovations
Moreover, the underlying principles of DeepSeek’s algorithms facilitate real-time data processing right at the edge. This capability is enhanced by cutting-edge hardware components like advanced processors and specialized sensors that contribute to overall efficiency. Customizable features such as energy consumption settings and connectivity options—including integration with 5G networks—further enhance its appeal. Additionally, scalability allows for seamless deployment across a variety of IoT environments, showcasing how DeepSeek R1 truly stands out in transforming capabilities within this space.
Exploring the Benefits of Edge Intelligence
- **Instant Decision-Making**: By processing data on-site, devices are able to make quick decisions without having to wait for information from far-off servers. This capability is essential for applications such as autonomous vehicles or safety systems in manufacturing plants that require immediate responses.
- **Enhanced Data Security**: Keeping sensitive information stored locally means it doesn't have to traverse various networks, similar to keeping a personal diary at home instead of sharing it publicly. This approach significantly boosts privacy and security.
- **Improved Network Performance**: Local data processing reduces the need for extensive internet bandwidth, making networks of interconnected devices more efficient and cost-effective.
Furthermore, the technical underpinnings of modern edge intelligence involve specific algorithms like federated learning and reinforcement learning that enhance its functionality. Additionally, specialized hardware components—such as dedicated AI chips and energy-efficient sensors—facilitate effective processing while minimizing latency and bandwidth use.
Real-world examples demonstrate how these technologies can transform various industries by improving efficiency and reducing operational costs while maintaining robust data privacy standards. These advancements illustrate the profound impact edge intelligence can have on Internet of Things (IoT) applications across different sectors.
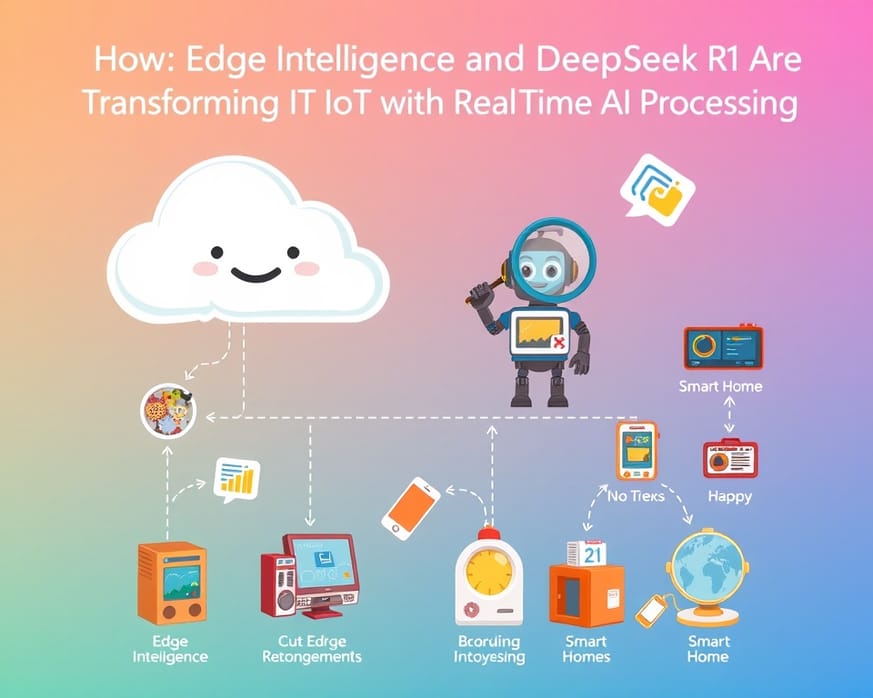
Key Technologies Behind Modern Edge Intelligence
## **Real-World Impact**
Edge intelligence is already having a significant effect across various industries. Here are some noteworthy applications:
1. **Healthcare**: Wearable technologies that incorporate localized AI can continuously monitor a patient's health and send alerts for any irregularities (such as changes in heart rate or potential seizures) without needing cloud support. This setup not only speeds up response times but also enhances privacy since sensitive health information remains on the device itself.
2. **Smart Cities**: Local processing of video feeds from traffic monitoring systems can help optimize traffic flow, minimize congestion, and boost safety—all without transmitting personal data back to centralized servers. This strategy alleviates network strain and mitigates risks related to data privacy breaches.
3. **Industrial IoT**: In manufacturing settings, machines equipped with edge AI can facilitate predictive maintenance by detecting possible failures before they happen. By analyzing sensor data locally, these systems enable equipment to take preemptive actions that help avoid costly downtimes.
Additionally, specific algorithms such as convolutional neural networks (CNNs) and reinforcement learning techniques play a crucial role in real-time data processing for these applications. Edge devices often come outfitted with GPUs or FPGAs that significantly enhance computational efficiency for AI tasks at the local level. Moreover, communication protocols like MQTT or CoAP optimize how data is transferred between devices, further streamlining operations in IoT environments where speed and reliability are paramount.
Real-World Applications of Edge Intelligence in Various Sectors
Despite the progress made by DeepSeek, there are still several obstacles to overcome:
- **Hardware Demands** - Even when models are compressed, they still require substantial computing resources compared to conventional edge processing solutions.
- **Model Effectiveness** - Although distilled models retain essential functionalities, there can be compromises when it comes to complex reasoning tasks.
Challenges Facing the Adoption of Edge Intelligence
Looking ahead, the future of Edge Intelligence appears quite promising, with several notable advancements on the horizon:
- **Enhanced Device Capabilities**: With improvements in AI model efficiency, even compact devices with limited resources will soon be able to execute sophisticated algorithms effectively.
- **Integrated Cloud-Edge Solutions**: We can expect a more seamless collaboration between cloud computing and edge systems, where both will complement each other by efficiently handling various components of AI-related tasks.
Overall, as technology evolves, we are likely to see smarter devices and better architectures that optimize performance while addressing concerns like latency, bandwidth usage, and energy consumption.
The Future Prospects for Edge Intelligence Technology
As emerging algorithms like federated learning and transfer learning continue to optimize real-time data processing, we can expect significant advancements in this field. Furthermore, the development of specialized AI chips and low-power sensors will enhance energy efficiency, making these technologies more accessible for widespread use.
The integration of 5G technology stands to greatly improve connectivity while reducing latency, ensuring that edge applications become more responsive than ever before. It's also crucial to focus on security measures specifically designed for edge environments to safeguard sensitive data during both processing and transmission. Altogether, these advancements provide a well-rounded perspective on the promising developments ahead in edge intelligence technology.
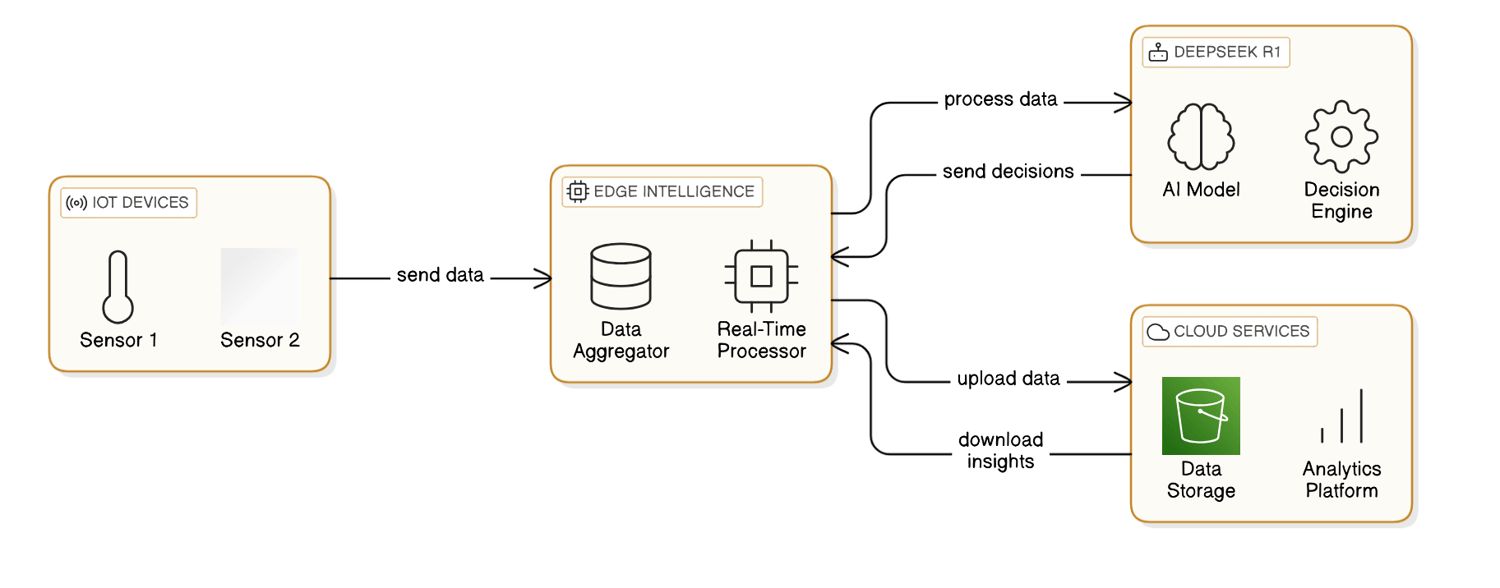
How Cloud and Edge Computing Will Collaborate
Conclusion on the Transformative Power of Edge Intelligence
Moreover, advancements in materials used for edge devices contribute significantly to their durability and energy-saving capabilities. For instance, the introduction of adaptive algorithms allows these systems to adjust dynamically based on fluctuating environmental conditions, further enhancing performance and reliability across various scenarios. As we look ahead, it’s clear that this synergy between cloud resources and edge computing will redefine how we interact with technology in our daily lives.
Reference Articles
Edge Intelligence: How DeepSeek R1 is Reshaping IoT's ...
Combining edge computing with efficient AI models like DeepSeek R1 offers three main benefits: Real-Time Processing — By handling data ...
Source: Medium · Thomas ClearyDeepSeek's implications for edge AIoT - Omdia - Informa
This report looks specifically at the implications of DeepSeek-V3 and -R1 models in the context of advancing the opportunities for edge AIoT ...
Source: OmdiaDeepSeek's open-source LLM brings AI automation and ...
DeepSeek's R1 model enables LLMs to run on edge devices, transforming AIOps and edge computing. This advancement enhances real-time insights, ...
Source: Edge Industry ReviewTransforming AI with DeepSeek
By combining deep learning algorithms with real-time processing power, DeepSeek R1 stands out as a leading-edge AI model, promising to redefine ...
Source: Medium · Manik RoyDeepSeek R1 is now available on Azure AI Foundry and ...
As DeepSeek mentions, R1 offers a powerful, cost-efficient model that allows more users to harness state-of-the-art AI capabilities with minimal ...
Source: Microsoft AzureDeepSeek's AI Innovation: An AI Model That Shift to ...
DeepSeek's ultimate goal is to achieve Artificial General Intelligence (AGI). The advancements in reasoning capabilities represent a major leap ...
Source: TechAheadEdge AI and the Future of Business: OpenAI o1 vs. ...
Key Insight: If real-time processing and low-latency AI are critical for your business, DeepSeek R1 is the better choice due to its open-source ...
Source: LinkedIn
Related Discussions